Articoli e interviste
18 Giugno 2018
"RAM Market-Neutral" Systematic, Fundamental, All-Cap Alpha. The Hedge Fund Journal profile interview with Emmanuel Hauptmann
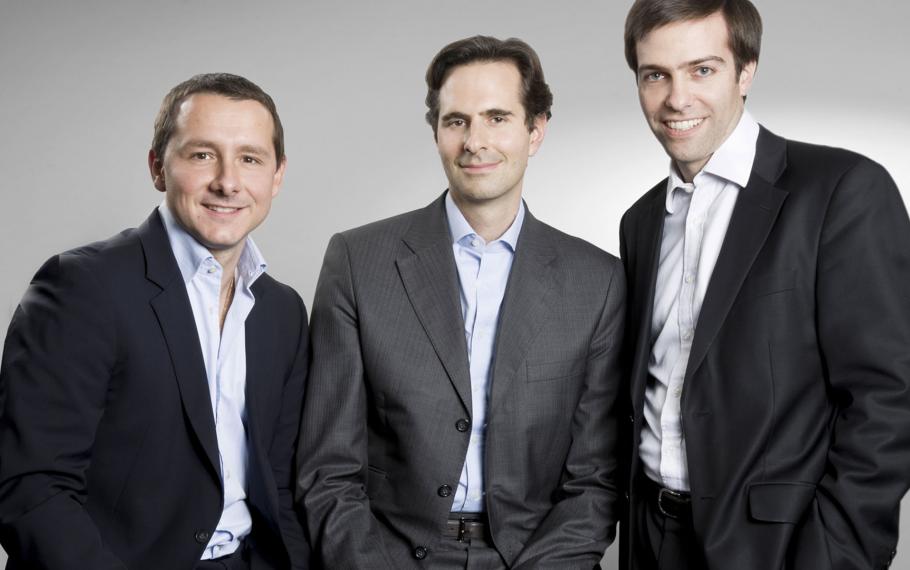

SOURCE: The Hedge Fund Journal - DATE: 08 June 2018 - MEDIA: Online - Hamlin Lovell
Geneva-headquartered RAM Active Investments (RAM) runs $5.1 billion as of 31st March 2018, with $1.5 billion in three quantitative, fundamental, equity market neutral strategies, and the rest in related systematic long only equity strategies, including an ESG strategy, and a separate discretionary/fundamental fixed income strategy.
The firm is now 69% owned by Italy’s Mediobanca, with previous owner Reyl retaining 7.5%. The three founding partners have significant personal investments in RAM strategies, and are all senior investment managers in charge of the investment process. The trio are Thomas de Saint-Seine, who joined in 2001 having been a portfolio manager at Credit Agricole Indosuez; Maxime Botti, who joined in 2004 having formerly been a risk arbitrage hedge fund manager at Equinoxe Partners and Barep; and Emmanuel Hauptmann, who joined in 2007, after a background in derivatives and quant at Citi and Morgan Stanley. The trio share responsibility for making all key investment decisions and for changing, improving and fine tuning the systematic equity models with the four other senior quants in the Systematic Equity team. “The seven of us carry out day-to-day research to improve our alpha generation and implementation. The team members are to some extent specialised into research areas such as liquidity, rebalancing, back-testing infrastructure, new data sources and new types of factors,” explains Hauptmann.
RAM received The Hedge Fund Journal’s UCITS Hedge Awards 2018 performance awards for best risk adjusted returns in two categories: Equity Market Neutral (Quantitative) – Emerging Markets over one and five years, and Equity Market Neutral (Quantitative) – Europe, over three, four and five years (for periods ending in December 2017).
RAM targets a Sharpe ratio of one, with through-the-cycle volatility of 8% for the European and global strategies, and 6% for the emerging markets strategy, which hedges currency risk.
Performance attribution
Performance attribution has been mainly single stock alpha. Around three quarters of performance for RAM long/short European equities – RAM’s oldest hedge fund strategy, launched in March 2009 – has come from single stock selection rather than country or sector positioning; (RAM’s systematic long only European equity strategy dates back to 2005). Country and sector net exposures can be as high as 25% or 30%, and are typically around 10-15%, but idiosyncratic security selection is also the key return driver for the other two equity market neutral strategies: RAM long/short emerging markets equities (launched in December 2011), and RAM long/short global equities (launched in December 2016). Realised beta has averaged near zero, though it can fluctuate between +0.2 and -0.2.
RAM has, on average, generated more net alpha from the long side, partly due to financing and stock borrow costs on the short side. RAM is not that unusual in finding that mid-caps are a sweet spot for alpha generation on the long side. But RAM is highly distinctive in generating significant short alpha from small cap stocks; many hedge fund managers have little or no short book exposure to small caps. “In distressed markets such as 2011, the short book has come into its own and generated more alpha, when it was harder to do so on the long book,” points out Hauptmann (as shown in Fig.2). For example, “single stock shorts helped our European long/short strategy to roughly preserve capital in 2011, which saw dislocated names, high beta and high volatility,” he adds. Portfolio protection during ‘risk-off’ phases is not without potential costs however, and shorts can lose money during ‘risk on’ episodes. RAM’s European long/short strategy saw its largest drawdown – of 10.9% – between December 21st, 2015 and April 22nd, 2016. Hauptmann recalls, “there was a mix of a cyclical rebound of commodity industrials, and large ECB intervention with an extra EUR 20 billion of asset purchases, creating a massive risk-on environment – particularly for lower quality, higher leverage, companies. The most volatile and high beta companies bounced back very quickly”. Indeed, this type of rally can be described as a “dash for trash”.
RAM Active Investments (RAM) runs $5.1 billion as of 31st March 2018.
In terms of broad styles, RAM has three proprietary models: value, defensive/low risk and momentum/GARP (growth at a reasonable price). The last has been the biggest contributor to the European equity strategy since inception, albeit with higher risk. But in some years, such as 2012 and 2014, RAM’s value models have made the most profit. RAM’s value models have generated lower returns overall but also with less risk, and have greatly outperformed generic value measures such as price to book value. In 2017, RAM’s value models even beat the long only European equity index, in a year that was challenging for traditional value approaches. Attribution by style is shown below.
RAM’s process is informed by a blend of academic research and proprietary modelling, based on RAM’s own experience of sifting through cash flow statements. “We make many adjustments to accounting statements,” reflects Hauptmann. For example, RAM’s value model is based partly on Fama and French’s 2011 research combining value and momentum, and also on RAM’s own measures of free cash flow, profits and balance sheets.
Incremental evolution
The three key styles have been present since launch and each one encompasses much more than their names might suggest. For instance, RAM’s value concept unsurprisingly requires strong free cash flow and profitability, but also includes a criterion that could be associated with the ‘quality’ factor: a strong balance sheet. Similarly, RAM’s momentum/GARP model is tempered with some value criteria, as is their defensive/low volatility model.
“The three models slowly and incrementally evolve with improvements, refinements and fine tuning, such as integration of new data. Changes are only made if they improve return and risk on an out-of- sample basis,” says Hauptmann. “Some changes have also removed biases or increased capacity,” he adds. The degree of change should not be exaggerated; return enhancements have typically improved hit ratios by a few percentage points, and increased Sharpe ratios by perhaps 10%. Whereas some systematic managers claim that older versions of their models are vastly inferior, RAM does not calculate a massive spread between the historical prototypes and its current model.
Future research
The future evolution of RAM’s research involves using deep learning algorithms to combine new data and factors. “The amount of data, and the number of factors, keeps growing, with data sources including financial statements, estimates, management transactions data, market databases of price, liquidity, risk, stock lending, and sentiment,” explains Hauptmann. “It becomes harder to combine the factors, so we need a deep learning approach, including artificial neural networks, to allow for a non-linear combination of factors and how they interact in a systematic manner,” he goes on. “As the most advanced technology is open source, we use it, but have developed our own back-testing infrastructure and algorithms,” he adds.
Fixed alpha weights
RAM does not move alpha weights around very much. Some managers attempt to time alphas and factors by varying model weights, but RAM maintains stable weights in order to emphasise bottom-up stock selection. “We believe that these fundamental inefficiencies persist through market cycles. At some phases of cycles, certain inefficiencies will underperform as they are overcrowded or out of fashion. But at least one of the engines is always working well over some stage of the cycle,” says Hauptmann. For example, value often does best in the first stage of the cycle, momentum/GARP in the second, and defensive/low volatility later on, when volatility picks up. For RAM, keeping stable factor weights is more about reducing risk than increasing returns. “Other approaches could become concentrated into certain inefficiencies and add risk versus a stable allocation. We think it is more optimal to have a stable and robust blend of engines,” explains Hauptmann.
Turnover and trading
Though keeping steady factor weights might seem like a default choice, the frequency of rebalancing intervals is an important active decision. As better performing alpha engines become a larger part of the portfolio, RAM needs to rebalance to maintain constant weights, in effect taking profits on, and top-slicing, the outperforming models and adding exposure to the under-performing models. Since this incurs transaction and liquidity costs, RAM’s proprietary market impact models, partly informed by academic research co-authored by Hauptmann, have determined that the optimal interval is six weeks, but there is no wholesale switch across the portfolio every six weeks. “The process is staggered, with each sleeve of the portfolio rebalanced on a different schedule, so that a small fraction turns over every few days. This captures and incorporates new information in a more continuous and smooth manner. The process actively and dynamically takes on board new data including analyst estimates, and new financial statement releases,” says Hauptmann.
Industry and sector exposure
RAM can have some industry and sector bets, which fluctuate substantially as relative valuations and fundamental data change. RAM’s net exposures are shown in Fig.3.
For instance, in March 2018, there was a net short position in technology, in both developed and emerging markets. “High levels of valuation in quite a few names create very attractive shorts for the strategies,” says Hauptmann, who adds: “there is much dispersion in technology sector valuations, so we tend to have decent long and short exposure in the sector”. Industrials are another sector where RAM’s models exploit diversity: “we find the full spectrum of companies, from high quality, well managed, strong cash-flow generators with good growth dynamics, to highly leveraged, shareholder-diluting companies,” expounds Hauptmann.
Differentiated short models
Short positions can be based on a variety of models derived from observed market inefficiencies. RAM uses some well-known market inefficiency models, such as the accruals anomaly, from Hirschleifer, Hou and Hong Teoh’s 2010 paper, to gauge quality of earnings or Frazzini and Pedersen’s 2011 low risk model, and these are complemented by RAM’s own research.
“Short EPS negative convexity looks for negative inflection points in earnings; short over investment seeks out companies with too much capital spending, and working capital or inventory relative to peers, often combined with low quality earnings; and the short value cycle model looks for mean reversion back to cyclical average valuations,” Hauptmann illustrates.
Some of RAM’s short models are conceptually the opposites of its long models, such as DASP (decay at stretched price, a new acronym coined by RAM), which is the opposite of GARP, while other short models are specific to the short book – and even specific to geographies. Short value cash flow and DASP apply to all three regional strategies but some other models, such as short value cycle, apply to two of the three: emerging markets and global. And a few models are used only in regions: short overstated earnings (inspired by research from Beneish and Nichols’ 2007 paper) is used in emerging markets; short negative convexity EPS in the global portfolio, and short over investment in the European strategy.
“The short side has more emphasis on low quality of earnings, financial transparency and potential overstatements of earnings, such as negative free cash flow versus cash flow from operations; firms with high leverage and strong equity issuance. This low-quality bias helps tremendously when the credit cycle turns, and during risk-off events in the market,” says Hauptmann.
ESG
RAM are among a growing number of alternative asset managers who are signatories of the UN PRI. All RAM strategies, including their hedge fund strategies, exclude from their long book companies involved in: anti-personnel mines, cluster munitions and weapons; ILO child labour; breaches of human rights and ethical norms; environmental damage; and those that treat minority shareholders badly. Some managers would also exclude such firms from their short investment universe, but “we are happy to synthetically short low ESG ranking stocks,” says Hauptmann. This naturally also means that RAM can use mainstream indices on the short side rather than dedicated ESG indices. In any case, a very small percentage of companies are excluded by these criteria.
RAM also has dedicated long only ESG strategies that will exclude a much larger percentage of the investment universe, such as companies involved with coal, tobacco, gambling, and those with large carbon footprints, based on data from CDP. RAM contemplates launching a market neutral version of their ESG strategy.
Small cap shorting
Part of RAM’s short book is implemented through broad liquid market indices, to maintain beta neutrality. The percentage of single stock shorts varies by region: it is 35% in emerging markets, 45% in Europe and 60% in the global strategy. Hauptmann explains “it is harder to source names for borrow in emerging markets whereas it is easier to borrow across developed markets”.
RAM finds that trading across all market capitalisations is optimal for risk adjusted returns.
Some hedge fund managers have a larger average market capitalisation on their long than on their short books, creating a size factor bias. While RAM does use some stock indices on the short side, its single stock short book has a sufficiently high weighting in small caps to eliminate “size bias”, according to Hauptmann. RAM’s small cap shorts have been most profitable, and RAM obtains stock borrow on around two thirds of intended shorts, thanks to using two prime brokers with very good inventory.
Capacity
The need to retain a significant small and mid-caps sleeve, on long and short books, defines strategy capacity constraints. The long books of RAM’s three long/short strategies very closely overlap with the corresponding long only funds and “each position is scaled according to its liquidity, bid/ask spread, and volatility profile,” says Hauptmann. RAM’s largest strategy, emerging markets long only, has soft closed at $2.5 billion, to maintain its small cap exposure; “paradoxically, the emerging markets strategy would recently have performed better with a large cap bias, but we believe that small and mid-caps are useful for long term diversification and alpha opportunities,” points out Hauptmann.
Gross exposure has been below 230% for the European equity market neutral strategy and less than 180% for the emerging markets one, which also hedges currency risk. RAM’s newest hedge fund strategy, the global long/short strategy, has capacity of $3 billion, while the European equity strategy has estimated capacity of $2 billion on the long side (it currently has assets of $500 million in long only and $800 million in long/short).
There is limited position and capacity overlap amongst the global, and European or emerging markets strategies, for several reasons. The global strategy has a minimum market capitalisation of $500 million, whereas the European and emerging markets strategy have minimum market capitalisations of $150 million. The global strategy also uses a different optimisation process for stocks above $500 million. Additionally, geographic overlap between the global and emerging markets strategies only occurs in Hong Kong and South Korea. The global strategy investment universe is 6,000 stocks (of which typically around 600 will be traded at any point in time); the emerging markets strategy will usually select 600 from 3,500 possible stocks, and the European strategy draws a similar number of stocks from a universe of 1,500.
UCITS and Cayman
Nearly 100% of RAM’s assets are now in UCITS vehicles, which are registered for distribution in over ten European countries, and in Singapore. Two Cayman vehicles remain, in the emerging markets long only and Europe long/short strategies, and these can accept investments from certain institutional US investors. “We have no managed accounts and have always persuaded investors to go into comingled vehicles, as we find they are better for implementation,” says Hauptmann.
The UCITS have a share class offering lower management fees for allocations above EUR 20 million, and this has attracted some pension fund and insurance allocations. All research costs including data have always been absorbed by RAM, so MiFID II has not changed this.