News
12 March 2020
Unlocking the Secrets in Semantics
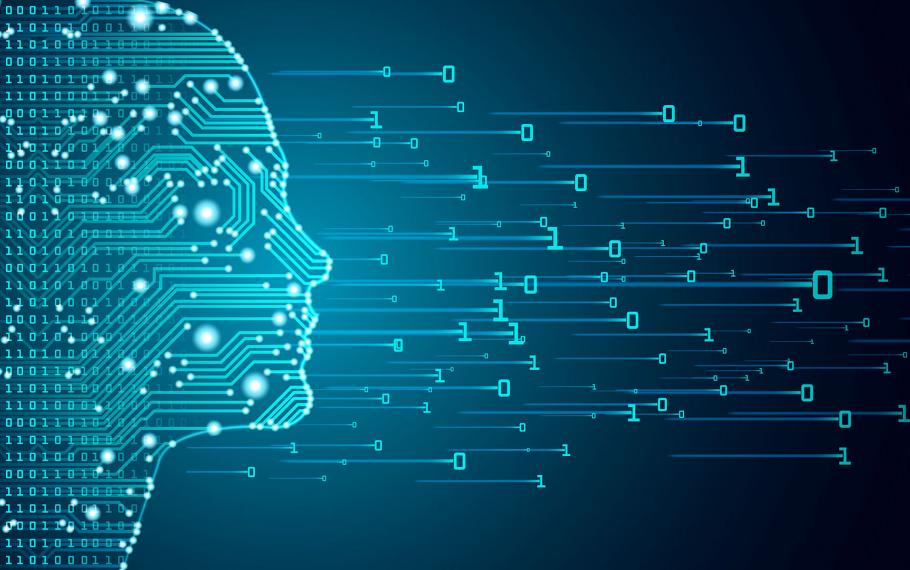
Natural Language Processing or “NLP” is a branch of Artificial Intelligence "AI", which aims to enable machines to be able to read, decipher, understand, and ultimately make sense of human language in a manner that is of value. NLP is increasingly automating operational processes ranging from the simple; answering a question from the internet, to the more complex; processing gigabytes of unstructured data, and generating terminologies, making implicit connections, and inferring that data’s context.
Today, NLP is the driving force behind some of the most commonly-used applications across our day-to-day lives:
- Language translation applications such as Google Translate
- Word Processors such as Microsoft Word and Grammarly that employ NLP to check the grammatical accuracy of text
- Interactive Voice Response (IVR) applications used in call centres to respond to certain users’ questions and requests
- Personal assistant applications such as OK Google, Siri, Cortana, and Alexa
The NLP community’s current focus is on exploring several key areas of research, including; semantic representation, machine translation, textual inference, and text summarization.
Certainly, the recent advancements in Machine Learning techniques have enabled data scientists to advance these techniques hand in hand. Data is being generated and captured at an exponentially increasing rate, and NLP is an important tool in our box to enable us to better understand what is happening across global markets.
What are the challenges of using NLP in Finance?
Specific to what we do (systematic investing), traditional market and factor data are typically structured in numerical terms and are relatively simple to use within the machine or deep learning models. However, despite the abundance of rich textual data taken from financial news, earnings reports, and transcripts and their correlation to markets, currently, quantitative managers rarely exploit this text data. This is in part because raw textual data is represented by its categorical and symbolic features, which presents a problem for quantitative models. However, one key NLP technique, which could help overcome this issue, is language representation (i.e. text embedding). This technique transforms text symbols into numerically digestible high-dimensional (i.e. several hundred or thousands) dense vectors, while importantly still preserving semantic closeness. At RAM AI, we have developed a deep learning model using text embedding, capable of consuming both factor and text data to help capture their interactions and subsequently their effects on the wider market.
Extract of our Research piece on Natural Language Processing to be released in the coming weeks. Do not hesitate to contact us for further details on the advancement of our research.