Articles & Interviews
27 April 2020
How to deal with the complexity of data according to RAM experts
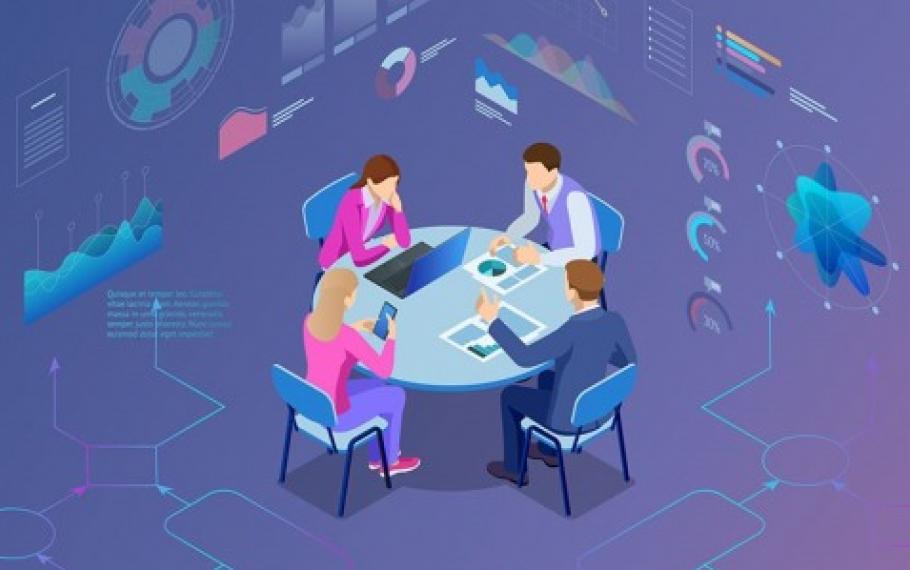
Citywire Switzerland by Camilla Giannoni
Artificial intelligence and machine learning technologies can be a useful tool for fund managers.
Senior quantitative analyst Nicolas Jamet and senior data scientist Tian Guo believe this is particularly true when they help combine different categories of data.
Talking to Citywire Switzerland, Jamet said: ‘We realised that the amount of data available was increasing exponentially. Integrating new metrics such as ESG into our existing set of information was becoming sub-optimal with traditional data analysis.’
Jamet and Guo separate them into two main categories: structured data and unstructured data.
Jamet said: ‘Structured data are numbers, pretty much anything that can be put into a table. Unstructured data can take the form of text, picture or video for example. The complexity comes from combining them and capturing the interactions between them. This is what machine learning, including natural language processing and deep learning techniques, helps us with.’
Guo said that to make text comparable with other data sets, the team uses natural language processing techniques. He said the process takes into account factors such as the type of news, the semantic meaning of such news and key words.
‘For example, when it comes to ESG, we aim to capture patterns between ESG related news and the market response of the companies. Trained algorithms analyse ESG news and aim to predict their impact in the current market.
‘We monitor news flows in real time and this allows us to incorporate timely information into our quantitative models of the company’s response.
‘On top of being fast, it is also objective because by integrating this data-driven process into the strategies we limit human interactions as much as possible,’ Guo said.
When it comes to ESG, Jamet believes having a process as objective as possible is key. He said the process integrates both historical and forward-looking data.
‘For example, we look at carbon emissions but we also want to know how the company is preparing for the transition to renewable energy sources. Ultimately, it is the algorithm which decodes non-linear interactions between these ESG metrics and traditional data sources,’ he said.