Articles & Interviews
3 April 2020
Wealth Net - Unlocking secrets in language helps investment process – expert comment
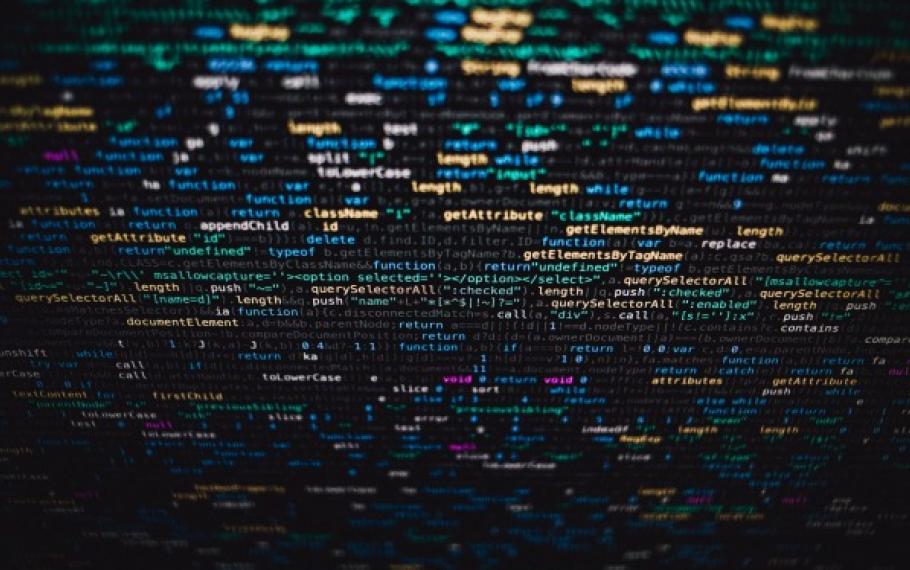
Wealth Net by Polina Hoare
Natural Language Processing (NLP) is a branch of Artificial Intelligence which aims to enable machines to read, decipher, understand, and make sense of human language in a manner that is of value.
To explain this, and how it can be used to benefit investment process, thewealthnet spoke to Tian Guo, senior data scientist at RAM Active Investments.
Quant specialist RAM AI is a Geneva-headquartered alternative investment manager with asset under management of $2.7 billion.
“The NLP community’s current focus is on exploring several key areas of research, including: semantic representation, machine translation, textual inference, and text summarisation,” explained Mr Guo.
“Certainly, the recent advancements in Machine Learning techniques have enabled data scientists to advance these techniques hand in hand. Data is being generated and captured at an exponentially increasing rate, and NLP is an important tool in our box to enable us to better understand what is happening across global markets.”
Being able to leverage NLP across real-time voice transcriptions and chat can provide additional data that can be integrated into RAM’s learning models. In terms of finance, NLP can become “a powerful tool” for asset managers to discover actionable insight from the realms of unstructured data that is produced throughout markets.
“Ultimately, we believe that the implications of NLP are profound and extremely positive for augmenting our current data sets for us to better-capture signals which can help us understand the markets in which we invest.”
The challenge of applying NLP to quantitative investment mainly lies in both data and model aspects.
Financial textual data comes from diverse sources, e.g. financial news, earnings reports, and transcripts, etc and consequentially is diverse in terms of data formats and structures.
The challenge here is how to transform these symbolic text data into model-friendly quantitative representations, in order to enable quantitative models to discriminate the semantic meaning in the text.
"Moreover, given quantitative representations, it is still nontrivial to design quantitative models on them. The financial text is relatively sparse, for instance, financial news moves in-parallel with real-world events (i.e. at sporadic times) and different types of news possess distinct relations to the market.
"Thus, it requires combining expertise both in machine learning and quantitative investment to tailor models to identify and capture genuine patterns. This process involves specialised model architectures, training techniques and so on."
At RAM AI, current NLP and Deep Learning efforts are two fold. First, the team applies text mining and NLP techniques to extract information from finance text (news, transcripts, earnings reports, etc.) and transforms them into quantitative “model friendly” features. Then, in order to boost its existing market prediction models, the team develops specialised deep learning architectures and learning procedures across both textual features and fundamental factors.
Mr Guo said that this will enable RAM AI to exploit the synergy of fundamental and text data for a more accurate prediction, and ultimately alpha generation.
“Moreover, our NLP and deep learning pipeline is generic and highly flexible, with the ability to adapt to different market segments depending on our interest.
"Second, to identify in real-time certain events or aspects of interest in the market by information extraction from diverse financial data. For instance, by plugging our in-house NLP pipeline into financial news flow, we can pinpoint company-specific ESG events and then inform clients in a timely manner.”
It is widely accepted, stressed Mr Guo, that real-world events reflected within unstructured data, e.g. financial news, earning calls, transcripts, financial reports, social media, etc, have a certain relationship to markets.
NLP enables inputs from these unstructured and qualitative data sources into quantitative models. These inputs, which are complementary to the firm’s existing quantitative/structured inputs from analysts’ revisions, enrich the information set that RAM’s quantitative models consume.
Meanwhile, with the quantitative models enhanced by unstructured data, the subsequent strategy selection process can react to real-time events and capture potential investment opportunities “in a more dynamic and timely manner”.
“For instance, more conventional climate and ESG related data are at a low frequency and data providers would typically take days or weeks to react, while automatically analysing news flow helps us to identify the latest ESG related issues on companies and assess their wider impact.”
Overall, the implications for NLP and deep learning techniques for money managers are profound, according to Mr Guo. If implemented correctly, their use expands the horizon of data in terms of variety, volume, and velocity, where variety is the type of data, volume represents the amount of data automatically processed, and velocity means one can process the frequently arriving data faster.
“These techniques can help to further automate quantitative investing. For these unstructured data typically processed by analysts before, we are now able to seamlessly integrate them into quantitative models with less human involvement, the associated inherent biases and time delays.
“For RAM AI, this automated pipeline does not infer the replacement of humans by AI. On the contrary, it serves to highlight the importance of domain knowledge and expertise in this field. This is because we still rely on domain knowledge to tailor the models, and to guide the algorithms and models to focus on important aspects of large-scale financial data.”
RAM Active Investments has offices in Geneva, Zurich, Milan and Luxembourg.